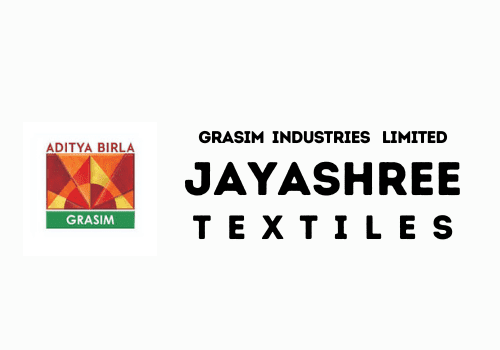
Jaya Shree Textiles is a unit of Grasim Industries Ltd. It was established in 1949 in Rishra, West Bengal. It has presence across Wool and Linen value chain. It is the largest, single location manufacturing facility in India for worsted yarn, wool tops. The largest integrated linen factory in India with latest spinning, weaving and finishing systems. Grasim Industries Limited, a flagship company of the Aditya Birla Group, ranks amongst the top publicly listed companies in India. It is a leading global producer of Viscose, Diversified Chemicals, Linen Yarn and Fabrics producer in India.
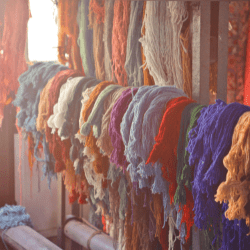
INDUSTRY
Textiles
COMPANY SIZE
$ 270 Mn
YEAR FOUNDED
1947
KEY USE CASES
Improve asset reliability by leveraging IoT and Artificial Intelligence (AI) for real time condition monitoring and fault prediction. Reduce the vibration analyst time spend on data collection and analyzing data.
PRODUCTS & INTEGRATIONS
ioEYE Predict Sensors
ioEYE Predict Predictive maintenance AI Cloud
Azure AD directory.
19%
Overall reliability improvement based on increased in MTBF & Uptime
Jaya Shree is the largest linen integrated facility in India. It has more than 42000 spindles and hundreds of machines – Hackling, Sliver Blending, Spinning, Power Looms (Weaving & Knitting), Dyeing and other balance of plant equipment. Jay Shree monitors conditions of bearings, gearboxes, fans etc across these machines by deploying ioEYE Predict vibration, ultrasound and temperature. They use AI powered fault prediction and reliability scoring to identify potential issues early, allowing maintenance to be scheduled before a failure occurs.
Challenges
- Downtime in textile industry can affect the entire production line. Lack of Insight into machine reliability and condition makes it challenging to take proactive measures and prevent unplanned downtime.
- Reliability baselining and corrective action threshold are not defined, this leads to subjective assessment of asset reliability. Specially balance of plant equipment like blowers, pumps etc. are neglected.
- The lack of digital records for asset health and breakdown data hinders the ability to recognize recurring problems and address them through root cause analysis and corrective action.
Outcomes
- IoT sensors monitor asset condition 24×7 and AI detects & predicts potential asset issues. This allows maintenance team to take proactive measures before any unplanned downtime occurs.
- With AI-powered Predictive maintenance software defining asset baselines utilizing historical performance and ISO standards for monitoring and targeting is easier.
- One can carry out entire asset fault analysis for a period of time. With this historical information it’s easier to carry out root cause analysis and identify assets that have recurring issues..
Revolutionizing Reliability: Unleashing the Power of Digitization 4.0 for plant maintenance.
Jaya Shree is the largest linen integrated facility in India and one of the largest linen spinners globally. With continuous expansion in the last two decades they have production capacity of more than 10 million metres of linen shirt fabric/ annum. With their state-of-the-art machinery and quality control system they have established themselves as a leader in the manufacturing of linen yarn and linen cotton fabrics. With this mindset of leadership and continuous improvement they wanted to improve their plant reliability, maintenance practice and reduce unplanned downtime.
They had a planned maintenance management system and wanted to augment it with a condition based maintenance system. Unplanned downtime, was a nightmare for Jay Shree. It lead to production schedules being disrupted and causing production loss. The team wanted to move to a more proactive approach to maintenance. Recognizing the need for a proactive approach, the Jay Shree team approached SenseGrow.
SenseGrow introduced a comprehensive cloud based condition and predictive maintenance system. The system enabled periodic condition monitoring using sensors and IoT devices. These sensors fed crucial data into AI algorithms, which meticulously analyzed trends and patterns.
The adoption of AI-powered predictive maintenance marked a turning point for Jay Shree textile factories. Machinery issues were diagnosed with detailed root cause analysis and data driven approach. This is leading to a remarkable improvement in maintenance outcomes and machine reliability. Moreover, AI-powered predictive maintenance has enabled their business to shift from reactive to proactive maintenance – with the ability to identify potential issues before they cause disruptions. This not only saves time and money but also enhances safety and reliability. The team is now empowered with the insights needed to optimize maintenance schedules based on this historical data. This has optimized their planned maintenance and reduce reactive maintenance incidents.
The predicitve maintenance solution provided by SenseGrow has proved to be helpful for our plant. It has automated our maintenance and also reduced the downtime for us. I recommend the product to people in maintenance.
Ashok Viveki
GENERAL MANAGER
Explore the products which help our customers grow
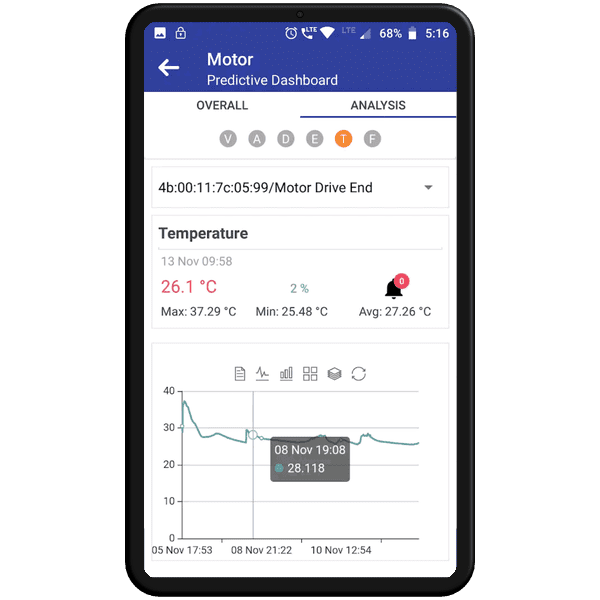
AI and cloud platform for predictive maintenance
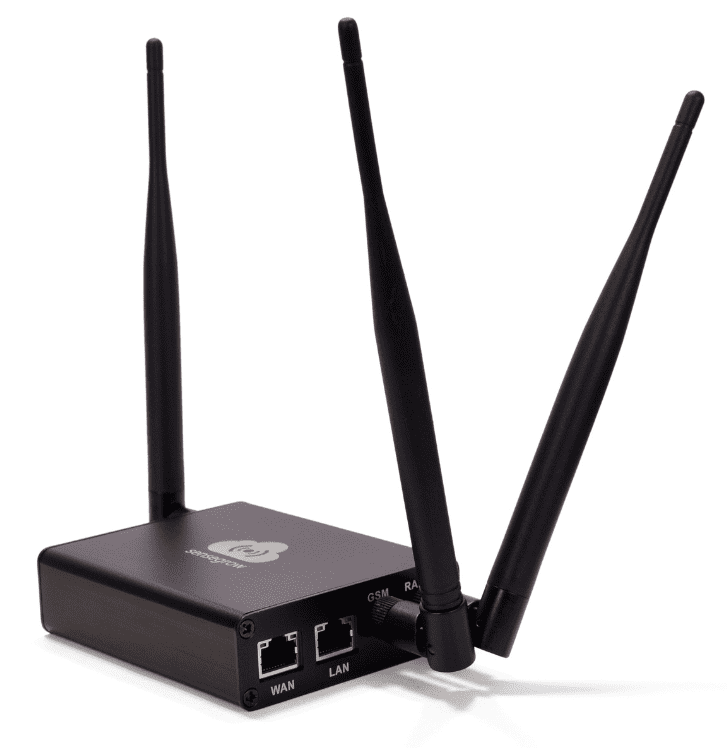
IoEYE Predict Gateway
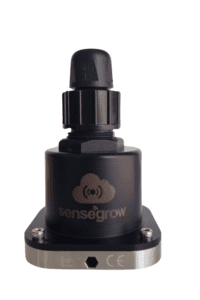